A great technology for oil and gas, utilizing Machine Learning to refine reserve estimates
Petroleum engineers invest much effort in estimating oil and gas reserves, and rightly so. The value of reserves is inventory for producers and a significant component of an oil and gas company’s balance sheet. As shown in the table below, five basic methods currently exist to estimate reserves.
The applicable method depends on the intended use of the information, the geologic setting, and the available data. The table below outlines these different methods and their applications:
Method | Type of Data Required | Applicability | Usability | Degree of Confidence |
Reservoir analogy | Recovery trend from other developed reservoirs in similar geologic settings | Any reservoir in the absence of “hard data” | The only method available at the earliest stage of the reservoir life cycle when sufficient data are not available | Low to moderate |
Volumetric analysis | Static data including reservoir pore volume, rock, and fluid properties; recovery efficiency | Any reservoir, from small to large if reservoir characteristics are known with some degree of confidence | Used in the early stages of reservoir life cycle | Low to high |
Decline curve analysis | Reservoir performance history including rate decline | Best suited for small fields where decline trends are clearly identifiable | Used when initial production data are available | Moderate to high when an appropriate decline model is used |
Material balance | Reservoir performance history | Small to medium-sized reservoirs where reservoir complexities are not predominant | Used when production data are available | Moderate to high in relatively simple cases |
Reservoir simulation | Detailed reservoir characteristics and production history | Appropriate for complex reservoirs having many producers and injectors | Used when ample reservoir and production data are available | Moderate to high when reservoir model is history matched |
Figure 1: Table of the five current methods of estimating hydrocarbon reserves. Source: “Conventional and Unconventional Petroleum Reserves – Definitions and World Outlook”, by Abdus Satter, Ghulam M. Iqbal, in Reservoir Engineering, 2016.
In typical conventional geologic settings, geophysicists and geologists in the geoscience community who work on seismic interpretation typically precede the work of petroleum engineers.
Seismic interpreters routinely analyze seismic data to find patterns that represent geologically important seismic facies. Seismic interpretation is essential in identifying and evaluating several key elements of a petroleum system, such as configuration for migration pathways, geometry and quality of the reservoir, the extent of compartmentalization, and seal capacity. Stratigraphic studies, fault detection and analysis, and horizon mapping support these objectives, and seismic attributes enable these results. These investigations are predicated on modern high-resolution 3D seismic surveys where prestack time and depth migration data enable geoscientists to interpret subsurface features related to structural and stratigraphic traps.
The culmination of the geoscience work processes enables petroleum engineers to do their important work. However, new machine learning technologies allow geoscientists to obtain a statistical estimate of reserves early in the seismic interpretation workflow. Geophysical Insights, for instance, reports using machine learning (ML) classification of multiple seismic attributes simultaneously to discriminate geobodies and quantify the volume of seismic features. Geobodies are defined from one or more ML neurons from stratigraphic analysis, seismic fault detection, or seismic facies classification. The output is a three-dimensional visualization of geological features, such as the channel feature highlighted in Figure 2. Geobodies produced through ML-based stratigraphic analysis or seismic facies classification – often a product of deep learning applied to seismic images – are 3D images of subsurface features.
A benefit of using ML in the seismic interpretation process is obtaining estimated volumetrics on potential reservoirs early to guide the balance of the geoscience analysis and engineering work to follow. Using the appropriate petrophysical parameters, such as velocity, net/gross, water saturation, and porosity, a relatively quick estimate of the hydrocarbon pore volume is practical for seismic interpreters. Once geobodies are isolated, the current state of ML-based seismic analysis tools enables geoscientists to do the following:
- Investigate geobodies at the sample level of each neuron
- Capture details on areas of interest, including volumetrics and statistics
- Edit selected geobodies by filling in spaces or pruning extraneous samples
- Export specific geobodies to an interpretation system for further analysis
Once generated, ML geobodies can also corroborate ML classification results to well logs. Geobodies resulting from fault detection are 3D views of fault surfaces (Figure 3), which inform the oil and gas drilling program. Seismic faults are drilling hazards, but faults can also compartmentalize the storage reservoir, so detecting faults is essential. And geobodies representing faults are more geologic – realistic – than traditional approaches that treat faults as planes.
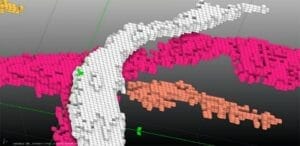
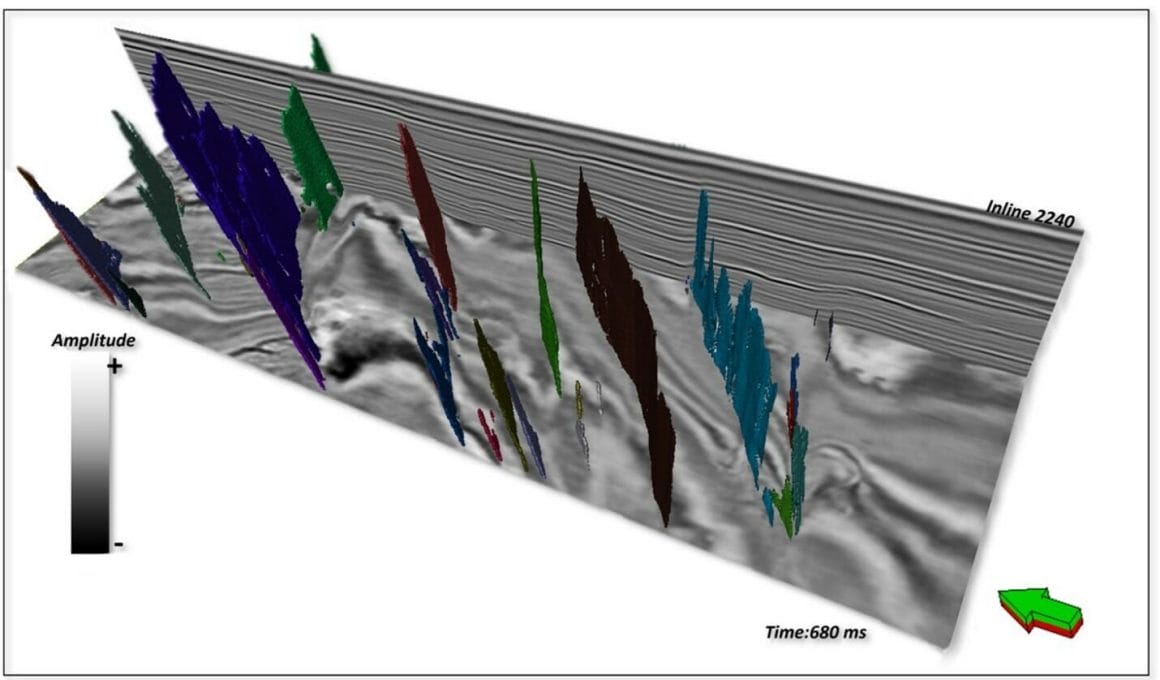
Conclusion
Geobodies can be derived from applying the SOM unsupervised machine learning method on multi-attribute classification. When this is done at the single-sample (voxel) scale, geobodies are then comprised of individual voxels. Whether the seismic data is in time or depth, the volume of each voxel can be estimated with a few petrophysical parameters, enabling early estimates of the hydrocarbon pore volume. Geobodies of particular interest to the seismic interpreter or engineer can then be compared to well logs to corroborate geobody composition.
In this way, seismic interpreters can identify commercially prospective reservoirs, potentially collapsing the time required for interpretation while collaborating with petroleum engineers before final reserve estimates are generated. Geobodies can also be generated on fault detection volumes, and seismic faults are another type of geobody that inform the drilling program.